IMath Webinar Series: John Paolo Soto and Dr. Renier Mendoza
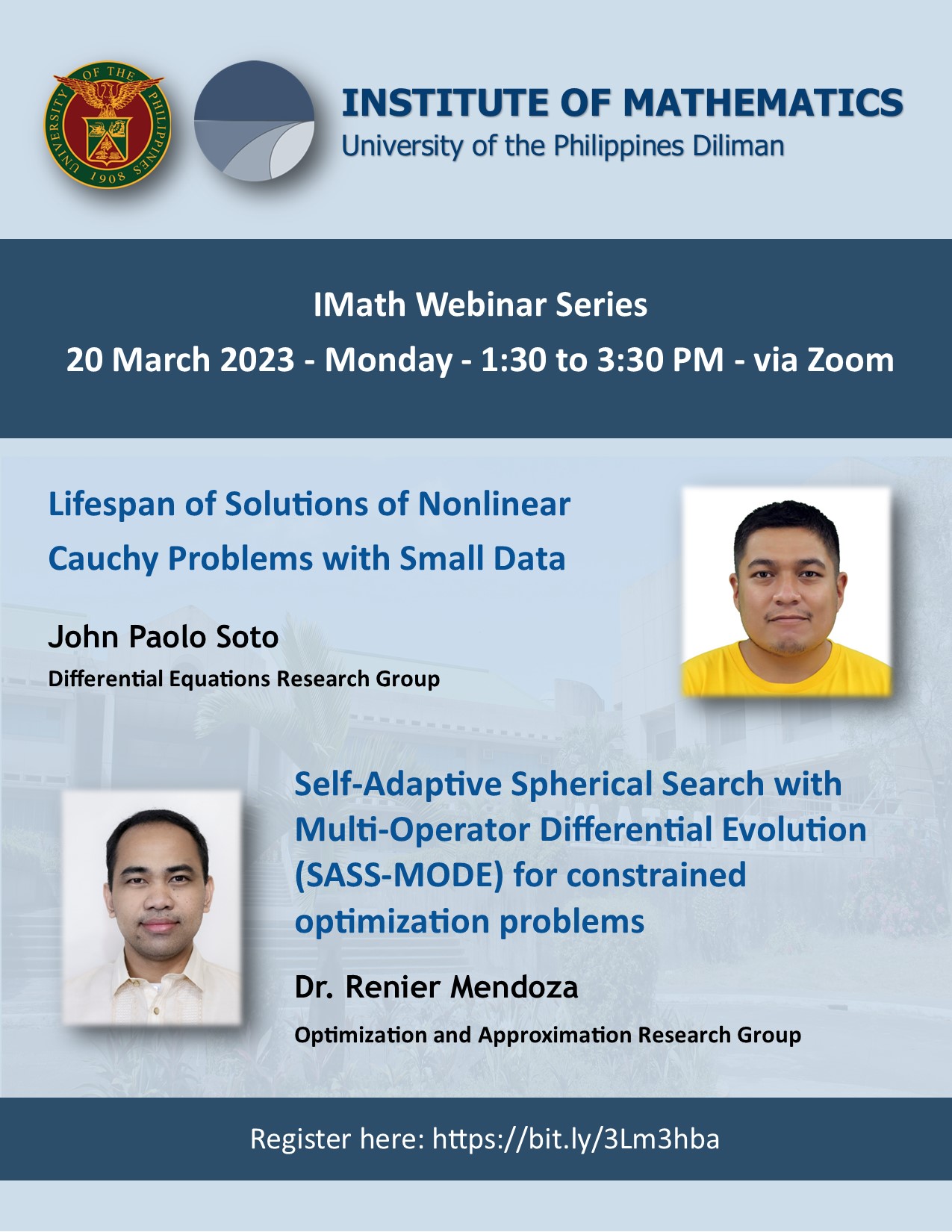
You’re all invited to attend the 1st installment of this semester’s IMath Webinar Series on Monday, March 20, 2023 at 1:30 PM. Mr. John Paolo Soto (of the Differential Equations Group) and Dr. Renier Mendoza (of the Optimization and Approximation Group) will be our speakers.
John Paolo Soto (Differential Equations Group)
Title: Lifespan of Solutions of Nonlinear Cauchy Problems with Small Data
Abstract: The lifespan of a solution, roughly speaking, refers to the largest possible value of
Dr. Renier Mendoza (Optimization and Approximation Group)
Title: Self-Adaptive Spherical Search with Multi-Operator Differential Evolution (SASS-MODE) for constrained optimization problems
Abstract: In this work, we combine a self-adaptive spherical search (SASS) with the improved multi-operator differential evolution to solve constrained optimization problems. SASS ranked first in the 2020 Special Session and Competitions on Real-World Single Objective Constrained Optimization, while the Improved Multi-Operator Differential Evolution (IMODE) ranked first in the 2020 Competition of Evolutionary Computation on Single Objective Bound Constrained Numerical Optimization. We have adapted IMODE to handle constrained problems by introducing three modifications: (1) hierarchical sorting for constrained handling, (2) a local search algorithm capable of solving constraint problems, and (3) a novel infeasible operator that allows population members to move to an “almost feasible” point with low cost.
Our hybrid method, referred to as SASS-MODE, is tested on 57 benchmark optimization problems from engineering applications and compared wit recent algorithms. Additionally, we apply our method to solve an optimal control problem arising from an infectious disease model.